World Cities Summit 2024
AI in Finance: Navigating Regulatory Challenges for Inclusive Growth and Resilience
Overview
The landscape of financial services has undergone a transformation driven by technological innovation. Developments in artificial intelligence (AI) and data sharing frameworks are redefining how financial institutions operate, creating new opportunities for consumers but also introducing significant challenges that need careful management.
SMU is studying the challenges of AI in finance led by Assistant Professor of Law Nydia Remolina Leon. The research explores three different themes and proposes some solutions.
Themes
Algorithmic Credit Scoring and Fair Lending
Imagine applying for a loan where your approval isn’t decided by a human but by a sophisticated AI system. This is happening right now through algorithmic credit scoring. To promote fair lending, emphasis is placed on designing better algorithmic models, having quality data, or even avoiding asking for specific information about the person (e.g., gender or race).
However, this research argues that controlling the inputs of the algorithm is ineffective in preventing unfair discrimination, as the effect of omitted characteristics (e.g., gender or race) may be reflected in other variables of AI models. Other approaches should be considered, including rigorous testing of credit scoring models for fairness, ensuring consumers understand how their data is used, and promoting equal treatment of traditional and alternative lenders. Additionally, the use of controlled environments or ‘sandboxes’ to safely generate and test data on typically excluded groups could further enhance fairness and inclusivity.
The Age of Generative AI
Generative AI (GenAI) can produce everything from convincing texts to intricate financial models, heralding new possibilities while introducing fresh challenges. The flipside includes legal and ethical challenges traditionally discussed in the field of AI governance, such as perpetuating bias hidden in training data while presenting new ones that require attention from policymakers, (e.g., improper prompt engineering). Criminals might also use these technologies to deceive through deepfakes.
One new notable issue with GenAI is ‘hallucination’, where AI fabricates plausible but incorrect or nonsensical information. This is especially concerning in sectors like finance, where precision and trust are crucial. Inaccurate AI-driven risk assessments or advice from AI advisors could mislead consumers. Addressing these ‘hallucinations’ demands meticulous model design, high-quality training data, effective prompt engineering, and safeguards such as fact-checking human oversight and feedback to maintain the reliability and integrity of AI applications. This research highlights the distinction between GenAI and predictive AI in finance, underscoring the need for discussions tailored to specific contexts in the financial services market, and proposes some solutions for regulators and the industry to consider.
Open Finance and Data Sharing Arrangements
The concept of open banking has allowed third-party developers to create innovative financial services by accessing bank data through Application Programming Interfaces (APIs). This has evolved into open finance that is driving greater innovation and competition. By providing AI systems with access to a more comprehensive dataset, open finance can significantly enhance the use of AI to learn more complex patterns, improve accuracy in predictions, and offer more personalised financial advice.
Different regions around the world have adopted varied regulatory approaches to manage the risks and leverage the opportunities of open finance. For instance, Singapore has adopted a market-driven approach with the regulator as the orchestrator of data sharing. In contrast, the European Union has adopted a mandatory approach in which banks and other financial institutions are required to share data of financial consumers with third parties. This research analyses these models and the differing national priorities and economic contexts they reflect, demonstrating that there is no one-size-fits-all solution.
Conclusion
It is vital for regulators and financial institutions to consider the broad societal impacts of AI in finance. Effective regulation and governance should not only address the inherent risks but also ensure that the benefits of AI in finance are widely distributed. Promoting transparency, protecting consumer rights, and fostering an environment of fair competition should be at the heart of future financial regulatory frameworks.
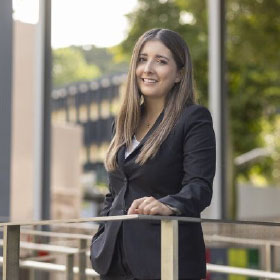
Lead Researcher:
Nydia Remolina Leon
Assistant Professor of Law, SMU Yong Pung How School of Law;
Head, Industry Relations, Centre for AI and Data Governance
Funding organisation: National Research Foundation
Read more about this study: